Like many others, we closely follow emerging trends in generative AI (Gen AI) and study how they influence user behavior across social networking platforms. This aligns with the objective we set for ourselves on day one — to provide a safe, inclusive, and innovative environment where people can connect and express themselves effectively. Below are a few of our observations, forecasts, research, and the decisions we've made, grounded in best practices — to protect our users and preserve the authenticity of their experience.
Forecast: Gen AI & Digital Spaces
Amid the rapid advancement of generative technologies, most experts agree: digital spaces are on track to become overwhelmingly saturated with synthetic content. In the context of the web, some forecasts even suggest that up to 99.9% of all content will be created by large language models (LLMs), for LLMs ↗. Unlike previous waves of automation, today’s agents not only generate content — they adapt to user behavior through reinforcement learning. This makes them nearly indistinguishable from real users and poses serious challenges for existing Web 2.0 platforms ↗
At the same we remain optimistic: while the ecosystem will inevitably evolve — with new, more adaptive and better-protected platforms emerging — the outcome will be net positive. Next-generation spaces will rise, grounded in transparency, agency ↗, and human connection.
Observation I: Assistants Enrich the Experience
Over the past few weeks, we’ve observed the launch of Grok as an assistant bot on X (formerly Twitter) — helping users interpret and verify facts in real time. We predicted the success of such solutions in TheEarth blog back in January, though we weren’t aware that X was planning a launch of this scale. By February, we had already introduced an interactive use case of our own.
Of course, not everyone — even among tech-savvy users — is comfortable with bots joining public discussions ↗. But we believe that, when used transparently, these assistants can enhance the digital experience in meaningful and constructive ways.
Observation II: Video Is a Pressure Point
Models like Google’s Veo 2 or Magi-1 from Sand AI, already make it possible to generate videos so realistic that even trained eyes struggle to distinguish them from real footage.
Yet video remains one of the most promising formats for detecting generative elements — because it combines visuals, audio, and timing patterns that enable both human and algorithmic identification.
Observation III: China’s Policy Approach
On March 14, 2025, the Cyberspace Administration of China released its final Measures for Labeling AI-Generated Content, taking effect on September 1 ↗. These rules require two types of labeling:
- Explicit labels: Visible indicators (text, audio, visuals) clearly informing that content was generated.
- Implicit labels: Embedded metadata containing details such as the provider’s name and content ID.
Additionally, platforms must implement systems that classify content as confirmed, possible, or suspected AI-generated, and reinforce these labels accordingly. This multi-layered approach reflects a growing global consensus: transparency in AI content is no longer optional — it's becoming a regulatory standard.
Our Point: Gen AI Is Not the Enemy
That said, we believe strongly: generative AI is not a threat — it’s a powerful tool. One of the most inspiring use cases is automatic speech dubbing. Just imagine when Antonio from a Naples pizzeria can share his passion for thin-crust pizza in 20 languages, all while preserving his tone, facial expressions, and gestures. That’s a cultural superpower — and a technology that brings people closer together.
Case Study: Trust, Disclosure, and Gen AI
Last week, we had a rare opportunity to closely observe how Gen AI affects the user experience — and what decisions we can make, responsibly and meaningfully, to improve it. We conducted a granular analysis, starting from the very first view, of the engagement evolution around a five-minute inspirational educational video, viewed by over 30,000 bilingual users. The respected speaker was real and well-known — and although his voice in the video was generated by AI, he personally approved the accuracy of the message. However, the fact that the voice was AI-generated was not initially disclosed to viewers.
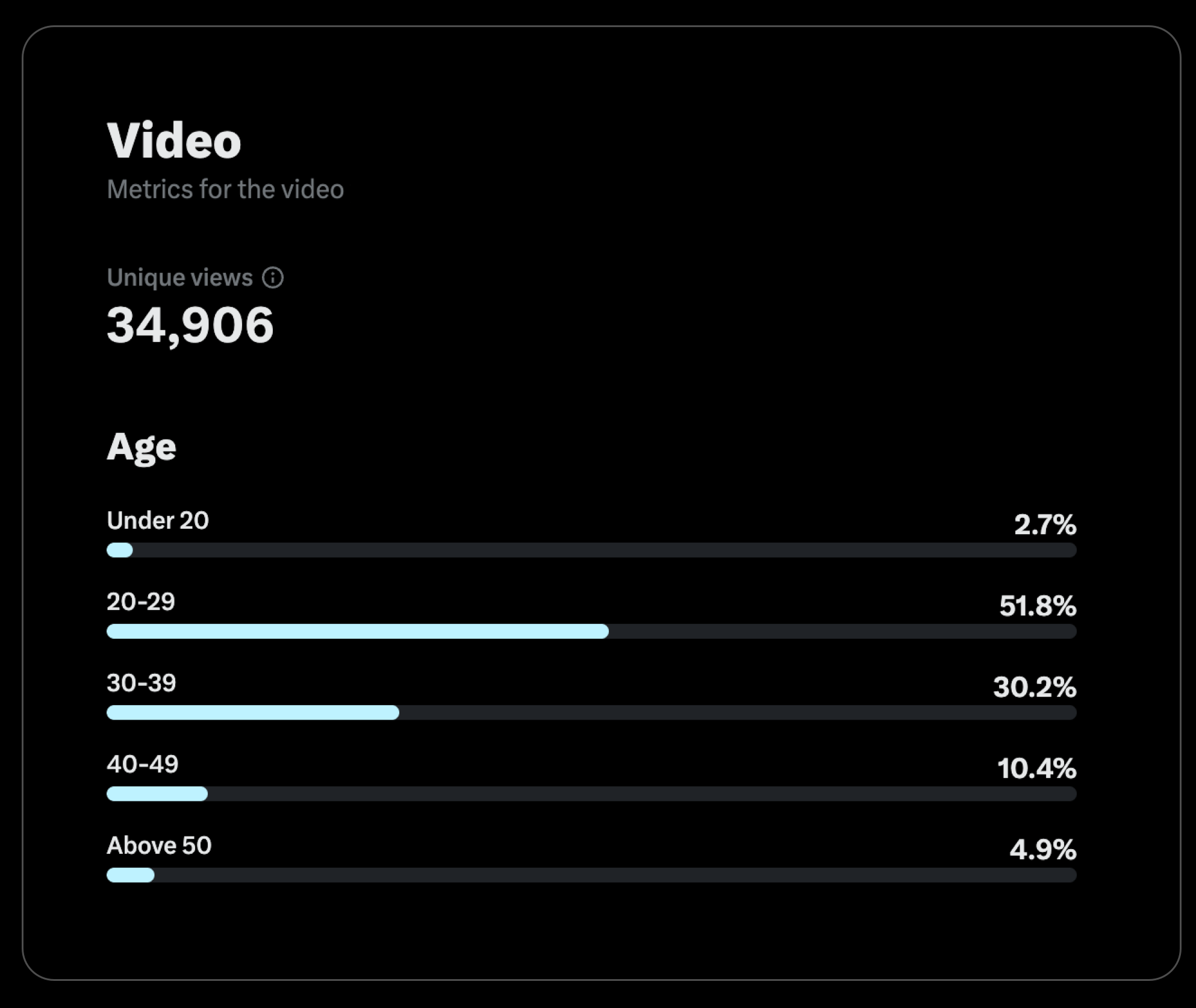
The voice sounded authentic — capturing the speaker’s intonations, emotions, and overall speaking style. The inspiring message and emotional tone were well received. Meanwhile, many viewers found themselves more focused on figuring out who was actually speaking — rather than staying with the message itself. Most viewers recognized the speaker, but reasonably assumed he was either speaking through a live interpreter or using some form of technology — as, in their view, he wouldn’t normally speak the language with the accent presented in the video. This sparked debates, with users questioning whether the entire video was authentic — and whether the speaker had actually said those words. Once viewers collectively clarified — and reached a consensus — that the video was faithful to the original message, it quickly gained viral momentum and received the recognition it genuinely deserved.
Our Takeaway
If the video had included transparent labeling — ideally, specifying which elements were AI-generated and originating from the speaker’s verified account — and had been proactively recognized by platform-level algorithms, viewer friction could have been reduced to nearly zero. Viewers would have been able to fully engage with the powerful and inspiring message, instead of being distracted by questions about its authenticity — while reasonably accepting minor imperfections in the auto-dubbing.
What We Learned
This analysis revealed a crucial insight:
Transparency of origin is more important than technical perfection.
Even though the AI-generated voice convincingly reproduced the speaker’s intonations, emotions and delivery style, the unfamiliar accent, combined with the lack of clear and trusted disclosure, led to temporary confusion and debate. Ultimately, it reduced the impact of the message until viewers themselves confirmed its authenticity. It showed that:
- People rely on contextual trust to process content — community, verified accounts, platform labeling.
- Authenticity is not just about realism — it’s about clarity of origin.
- When trust structures are missing, even inspiring and well-crafted content can lose its power.
And while these findings might feel expected, the real insight was this:
Summarizing, the study showed that when AI-powered communication is paired with transparent signaling and platform-level support, it can be incredibly effective. And this resonates with the words of one of the most sought-after teachers of our time:
"People don’t want you to be perfect. What they want is to feel connected to you."
Joe Hudson
Our Decision
Based on best practices, our research, and consultation with AI ethics experts, we’ve made a decision:
Any media content containing AI-generated elements must be transparently labeled and include metadata identifying its origin.
This isn’t a limitation — it’s a foundation for restoring trust in AI-generated content and fostering a standard for a healthier, more productive, and more honest information environment.
Writing headlines with the help of a language model? Makes perfect sense. Reaching global audiences in their native language with AI support? That’s inspiring. But users have an undeniable right to know what they’re engaging with. That’s why we’ve referred to them as conscious recipients.
Moving Forward
As we move forward, we’re actively exploring multi-layered solutions that improve transparency without limiting creativity or reach. Among the tools we’re evaluating are GenConViT↗
, StyleFlow↗
, and FTCN↗
— promising open-source models that may help ensure clarity and trust across video-sharing platforms.
And guiding us forward are the simple values:
We don’t optimize for attention. We optimize for authenticity, productivity, and human connection.
Research Limitations
Our studies have several important limitations to keep in mind when interpreting the findings. First, the results have not yet been peer-reviewed by the broader scientific community, meaning that they should be interpreted cautiously. In addition, the studies were based on observational analysis of engagement evolution in response to a specific speaker on a specific platform. Users engaging with different speakers or across other platforms may demonstrate different patterns or outcomes. Furthermore, meaningful shifts in user behavior and well-being may require longer observation periods to be reliably detected. As one of the engagement classifiers, we used view duration to reason about affective cues in user behavior. While useful, this metric is inherently imperfect and may overlook important emotional or cognitive nuances. Finally, our research focused exclusively on bilingual participants, underscoring the need for further studies across a broader range of languages and cultures to fully capture the complexity of emotional interaction in digital environments.